The ChatGPT-ification of Healthcare
AI can now navigate the nuances of conversations and describe complex images, unlocking many improvements in healthcare.
While AI seems like a new thing, the first AI programs for healthcare were created as early as the 1970s.
In 1972, Stanford University created MYCIN for bacterial infection diagnosis. MYCIN took in reported symptoms and medical test results, could suggest additional laboratory tests, and would come up with a diagnosis and a course of treatment. It had the same level of competence as human specialists in blood infections—and was better than general practitioners! Despite that, clinicians resisted it.
MYCIN isn’t exactly like the ChatGPT you know nowadays. It was known as an “expert system” made up of 500 if-this-then-that rules to help come up with the diagnosis. It would struggle with cases outside of these pre-defined rules. Given its limited value and resistance from the healthcare community, it was never widely adopted.
FDA-approved AI since 1995
But AI has been making headways into healthcare, even though most of us are not aware of it.
I was pleasantly surprised to discover that the U.S. Food and Drug Administration (FDA) has authorized 950 artificial intelligence or machine learning-enabled medical devices. And the number of approved devices has been increasing dramatically: 3 in the 1990s, 8 in the 2000s, 210 in the 2010s, and 729 in the 2020s (which is just five years so far!)
The first AI/ML-enabled device to be approved was the PAPNET Testing System, which analysed Pap smear slides to detect cervical cancer. It was approved in 1995—almost 30 years ago!1
Here are a few other notable FDA-approved AI:
Aidoc Briefcase (2018), the first AI for assisting radiologists, can detect brain bleeds in head CT scans.
IDx-DR (2018) can identify diabetes-related eye problems from images of the eye without needing any input from a specialist.
Viz.AI Contact (2018) can spot potential stroke in seconds and automatically inform a specialist.
EchoGo Heart Failure (2022) can pick up a specific type of heart failure, which is missed in up to 75% of cases.
Besides diagnostic tools, which required FDA approval, AI has been used in several other areas of healthcare:
Insilico Medicine uses AI to discover new drugs.
Tempus uses AI to personalize treatments.
Biofourmis uses AI to remotely monitor at-home patients.
(Fun fact: Swee Kiat used to work on using AI to predict protein structures at Stanford.)
ChatGPT for healthcare
So what’s next for healthcare, given the recent explosion of AI, with the most striking example being ChatGPT?
A lot of attention has been on using AI for diagnosis and rightfully so. If we can diagnose more accurately or spot illnesses (such as cancer) earlier, it can mean the difference between life and death. I hope we create even better AI in this area.
But healthcare goes beyond diagnosis and treatment. For patients, much of the frustration stems from poor experiences: navigating through complex insurance claims, enduring long waits at the clinic, or simply trying to reach a human on the phone. On the other hand, doctors are often overloaded by excessive administrative hassle that may not even be related directly to patient well-being.
The latest wave of AI, commonly known as generative AI, is different from its predecessors. Besides finding patterns in images or numbers, it can understand prose and images. Just like humans, it can handle the nuances of conversations and describe images, which unlocks many innovations.
A senior radiologist we spoke to shared that he often has to create long reports based on the medical images. He felt that large-language models (like those that power ChatGPT) can act as a junior radiologist who helps create preliminary reports. This then allows him to simply review and approve the reports. To him, this will truly make him more productive than current AI models that simply assist his diagnoses, something he has been trained to do. Medical imaging is often requested by clinicians as part of a broader diagnosis. Faster report generation could help these clinicians get their requested results sooner and treat the patients earlier.
Let’s take a look at a few more innovative applications of generative AI in healthcare:
1. Clinical documentation: Notes, referral letters, insurance claims
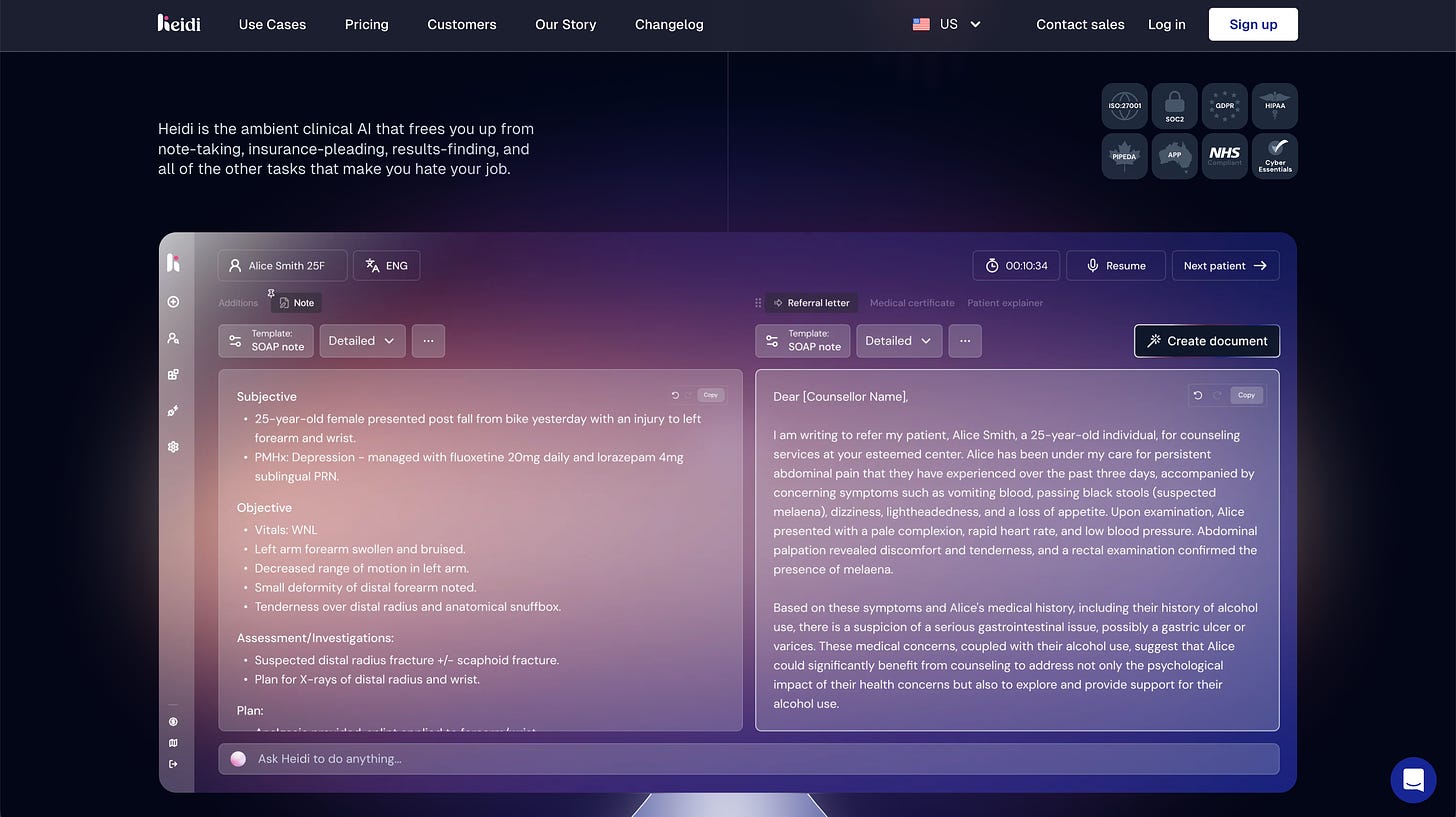
Clinicians used to have an actual human sit physically in their clinics or virtually via a camera to transcribe their consultation. Given its ability to understand conversations, AI is a great solution to this. Interestingly, radiologists have already been using speech-to-text software for decades. Because this is an obvious use case, many have jumped on this.
National University Health System, a major healthcare group in Singapore, has developed RUSSELL-GPT for summarizing patient case notes and writing referral letters in seconds. Our friend Dr Nelson Lau, a general practitioner for 30 years, is also tackling this at his startup Healthbridge. AWS also provides an API for transcribing patient-clinician conversations and generating clinical notes.
2. AI healthcare staff
To tackle the staff shortage crisis, Hippocratic AI created a marketplace of generative AI assistants. For now, they focus on low-risk, non-diagnostic, patient-facing tasks. For example, Linda is an AI agent who follows up with congestive heart failure patients by asking about their symptoms, medication adherence, and lifestyle adjustments. Instead of following a pre-written script, Linda can engage the patient, answer questions, and provide tips, similar to an actual nurse.
Hippocratic AI also has AI agents for checking in with patients before operations, advising on nutrition, helping to fill out health risk assessments, and more.
3. Medical copilots: Personalized screening and care plans
Early detection and treatment of cancer can drastically increase the survival rate and decrease the medical cost for the patient. According to the American Cancer Society, 99 out of 100 Stage 1 breast cancer patients survive for at least five years when treated early.
But many people don’t get screening in time; sometimes because their doctors don’t have the time or knowledge to get them to do the right screening. Even after the diagnosis of cancer, it can take days to weeks to complete additional tests to decide on the treatment.
Color, partnering with OpenAI, created a copilot to resolve these two issues. One, it can automatically understand a person’s background and recommend a personalized screening plan. Two, it helps primary care doctors and nurse oncologists know what additional tests are necessary so that they can immediately get started, saving patients weeks of wait.
4. AI doctor in our pockets
When I’m sick, I might not always want to go to a doctor because I don’t want to wait, I think it’s not too serious, and so on. Thankfully, my wife is a doctor, so I can call her. But most people don’t have medical professionals to call. According to a study in 2020, about 15% of people search for information about their illness before receiving a formal diagnosis from a healthcare professional. Given how Google results are filled with business content optimized for search engines, this feels dangerous.
Ada, created by a team of doctors, uses AI to understand your symptoms, suggest potential conditions, and give you trusted medical guidance. Perhaps the future of having a doctor in our pockets is not that far away!
5. AI-enhanced patient handoff
Before hospital doctors and nurses end their shift, they would inform the doctors and nurses for the following shift about the patients’ conditions and whether there are any follow-ups required. Depending on the hospital, this could be an electronic report or a physical paper note. And mistakes tend to occur because key information is neglected or forgotten. Sometimes remembering everything feels like an impossible task because nurses have to look after as many as 10 patients each.
Y Combinator (YC) startup Kabilah employs large-language models to automatically create digital reports from the patient’s electronic health reports and improve patient handoff. Google is also testing a similar generative AI technology at HCA Healthcare hospitals.
6. AI receptionist: Appointment scheduling and reminders
When we spoke to clinic assistants, we realized they have so much to do. They dispense drugs, help patients schedule appointments, handle insurance paperwork, and manage the inventory. It’s not surprising patients often have to wait on the phone just to ask a question or reschedule their appointment.
Startups like Vocca and Arini are hoping to change that with their AI receptionists. They can answer questions, schedule appointments directly into the clinic management software, and even keep track of patient’s requests (such as to review blood test results)—all without the monotonous robotic voice of automated phone systems.
7. AI call assistant: Credentialing, prior authorization, billing disputes
It sounds silly but hours and hours of healthcare professionals’ time are wasted on phone calls with insurance and pharmaceutical companies. For billing enquiries, please press 1, anybody? It can take seemingly forever to press 1 and 2 and hold on the phone to even reach a human to talk to.
YC startup Revia created an AI call assistant to not only navigate the automated menu and wait on the phone for healthcare professionals but also get prior authorisations and handle billing disputes.
“There is much more to health care than algorithms.”
While there is so much to be excited about, the quote above is a good reminder from Michael Abramoff, an eye doctor and computer scientist at the University of Iowa Hospitals and Clinics. He was commenting on Google Health’s project to test its AI tools in a real clinical setting. In a perfect setting, their AI could identify diabetes-related eye problems from an eye scan with more than 90% accuracy. But in the chaotic real world, where nurses have to scan dozens of patients every hour in poor lighting conditions, more than 20% of the images could not be used by the AI.
From our conversations so far, we have also heard similar advice from healthcare professionals and health tech founders. Technology is important but it is just a tiny part; understanding how it will be perceived and used in the real world is critical. All too often, well-intended AI solutions create more problems than they solve.
Let’s be mindful of this and build with clinical partners as we go about exploring new AI solutions.
P.S. What innovative AI applications in healthcare did I miss? Let me know in the comments!
For those curious, the PAPNET Testing System used a feedforward neural network.